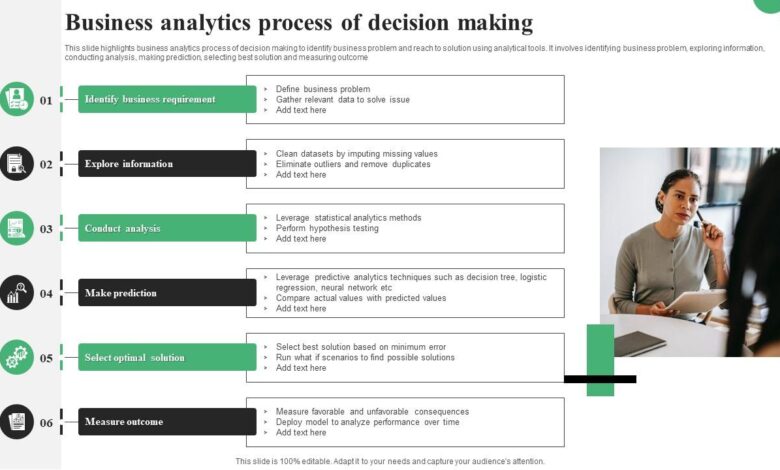
Business Analytics and Decision Making Quiz Master Data-Driven Decisions
The business analytics and decision making quiz dives deep into the fascinating world of using data to make better business choices. We’ll explore the fundamental concepts, practical techniques, and crucial tools that empower businesses to leverage data for improved strategies and outcomes. From data collection and preparation to analysis and decision-making processes, this comprehensive guide will equip you with the knowledge to navigate the complexities of modern business intelligence.
This quiz will cover everything from defining business analytics to applying various analytical methods in different business functions. We’ll examine the critical steps in the decision-making process, focusing on how to transform analytical insights into actionable strategies. Real-world case studies and examples will demonstrate the practical application of these concepts and highlight the transformative power of data-driven decision making in diverse industries.
Introduction to Business Analytics and Decision Making
Business analytics is the process of using data, statistical algorithms, and technology to understand and analyze business performance. It’s about extracting insights from data to drive better decision-making and ultimately, improve business outcomes. This involves examining historical data, identifying trends, and projecting future performance to make strategic choices. This process helps businesses optimize operations, personalize customer experiences, and make more informed decisions in a dynamic market.Business decision-making is a critical function in any organization.
Effective decisions require a clear understanding of objectives, consideration of potential risks and opportunities, and a structured approach to evaluating alternatives. Decisions impact all aspects of a business, from resource allocation to product development and marketing strategies. Successful business decisions lead to enhanced profitability, increased efficiency, and a stronger competitive position in the marketplace.
Relationship between Business Analytics and Decision-Making
Business analytics plays a crucial role in supporting decision-making by providing data-driven insights. Data analysis helps identify patterns, trends, and correlations that might not be apparent through simple observation. By leveraging this data-driven approach, organizations can make more informed and strategic choices, leading to better outcomes. This symbiotic relationship empowers businesses to make proactive decisions rather than reactive ones.
A Simple Framework for Data-Informed Decisions
This framework illustrates how data informs decisions in a business context. It’s a cyclical process that starts with defining a business problem or question. The next step is collecting relevant data, followed by cleaning and preparing the data for analysis. The analysis stage involves applying statistical methods, algorithms, or other techniques to uncover insights. Once insights are gained, they are used to formulate recommendations for action.
Finally, the impact of the decision is evaluated and the process is iterated to refine future decisions.
- Defining the Business Problem: Clearly articulating the problem is the initial stage. For example, a retailer might want to understand why sales are declining in a specific region. This initial step ensures that the subsequent data collection and analysis efforts are focused and relevant.
- Collecting Relevant Data: This involves gathering all necessary data points that can provide insight into the problem. For instance, the retailer would collect data on sales figures, customer demographics, competitor activities, and economic conditions in the target region.
- Data Cleaning and Preparation: Raw data often needs to be cleaned, transformed, and prepared for analysis. The retailer might need to handle missing values, inconsistent data formats, and outliers in the collected sales figures.
- Data Analysis: Statistical methods, data mining techniques, or predictive modeling can be used to analyze the prepared data. The retailer might use regression analysis to identify correlations between sales figures and economic indicators, or cluster analysis to segment customers based on their purchasing behavior.
- Formulating Recommendations: The insights derived from the analysis are used to formulate actionable recommendations. The retailer might recommend adjusting pricing strategies, targeting specific customer segments, or altering marketing campaigns to increase sales in the target region.
- Evaluating the Impact: The final step involves evaluating the effectiveness of the implemented recommendations. The retailer would track sales figures and customer responses to assess the impact of the changes. The evaluation data is then used to refine the framework for future decision-making.
Key Concepts in Business Analytics
Business analytics is transforming how organizations make decisions. It leverages data and analytical methods to uncover hidden patterns, predict future trends, and optimize business processes. This approach moves beyond simple data collection and interpretation, empowering companies to make data-driven decisions with confidence.Data is the lifeblood of business analytics. Understanding the types of data available and the analytical methods used is crucial for extracting meaningful insights.
Different analytical techniques are tailored to different business needs, allowing for a range of applications.
Types of Data Used in Business Analytics
Understanding the different types of data is essential for successful business analytics. This knowledge allows analysts to select the most appropriate analytical methods and avoid drawing incorrect conclusions. Data can be categorized into various forms, each with its unique characteristics.
- Structured Data: This type of data is organized in a predefined format, typically stored in relational databases. Examples include customer transaction records, product inventory information, and financial data. Structured data is easily processed by analytical tools and is often used for descriptive and predictive analytics.
- Unstructured Data: This type of data lacks a predefined format and is often found in text documents, images, audio, and video. Examples include customer reviews, social media posts, and sensor data. Extracting insights from unstructured data often requires specialized techniques like natural language processing (NLP) and machine learning.
- Semi-structured Data: This type of data falls between structured and unstructured data. It has some organizational structure, but not as rigid as structured data. Examples include emails, JSON files, and XML files. Semi-structured data often requires pre-processing steps before it can be used in analytics.
Analytical Methods Employed in Business Analytics
Business analytics utilizes various analytical methods to derive insights from data. Choosing the appropriate method depends on the specific business problem and the type of data available. Different methods provide varying levels of insights and forecasting capabilities.
- Descriptive Analytics: This method focuses on summarizing and describing past data. It answers the question “What happened?”. Examples include sales reports, customer demographics analysis, and website traffic analysis. Descriptive analytics provides a foundation for understanding historical trends and patterns.
- Predictive Analytics: This method uses historical data to predict future outcomes. It answers the question “What might happen?”. Examples include forecasting sales, identifying customer churn risk, and predicting equipment failure. Predictive analytics relies on statistical modeling and machine learning techniques.
- Prescriptive Analytics: This method goes beyond prediction by recommending actions to optimize outcomes. It answers the question “What should we do?”. Examples include optimizing pricing strategies, scheduling production, and personalizing customer recommendations. Prescriptive analytics uses optimization algorithms and simulation techniques.
Applications of Analytical Methods in Business Functions
Different business functions leverage analytical methods to achieve specific objectives. The application of analytics varies depending on the nature of the business and the specific challenges it faces.
- Marketing: Predictive analytics can identify high-value customers, segment customers based on their purchasing behavior, and personalize marketing campaigns. Prescriptive analytics can optimize pricing strategies and allocate marketing budgets effectively.
- Finance: Descriptive analytics can identify fraudulent transactions and detect unusual patterns in financial data. Predictive analytics can forecast future cash flow and assess credit risk. Prescriptive analytics can optimize investment portfolios and manage financial resources.
- Operations: Predictive analytics can identify potential equipment failures and optimize production schedules. Prescriptive analytics can optimize supply chain management and improve inventory control.
Comparison of Analytical Techniques
The table below summarizes the key differences between descriptive, predictive, and prescriptive analytics.
Technique | Focus | Methods | Applications |
---|---|---|---|
Descriptive Analytics | Summarizing past data | Data aggregation, data visualization, reporting | Sales reports, customer segmentation, website traffic analysis |
Predictive Analytics | Forecasting future outcomes | Statistical modeling, machine learning, data mining | Sales forecasting, customer churn prediction, equipment failure prediction |
Prescriptive Analytics | Recommending actions | Optimization algorithms, simulation, decision rules | Pricing optimization, production scheduling, supply chain optimization |
Data Collection and Preparation
Data collection and preparation are crucial steps in any business analytics project. They lay the foundation for accurate analysis and insightful decision-making. The quality and reliability of the data directly impact the validity of the conclusions drawn from the analysis. Thorough collection and meticulous preparation ensure that the insights gleaned are meaningful and actionable. Effective data collection methods, combined with careful data cleaning and transformation, pave the way for robust and reliable business decisions.
Data Collection Methods
Collecting data for business analytics involves a variety of methods. The choice of method depends on the specific business needs and the type of data required. Direct observation, surveys, experiments, and data mining are some common techniques. Each method has its strengths and weaknesses, and the best approach often involves a combination of methods to gain a comprehensive understanding of the business problem.
Data Cleaning and Transformation
Data cleaning and transformation are essential for ensuring the accuracy and consistency of the data used for analysis. Raw data often contains errors, inconsistencies, and missing values. These issues can significantly affect the results of the analysis, leading to incorrect conclusions. Cleaning and transformation processes help to identify and address these issues, resulting in a more accurate and reliable dataset.
Data Quality Issues
Data quality issues can significantly impact the reliability of business analytics results. Common issues include inaccuracies, inconsistencies, missing values, and duplication. Inaccurate data can lead to flawed conclusions, while inconsistencies can make comparisons and trends difficult to interpret. Missing data can lead to incomplete analyses and skewed results. Duplication of data can lead to over-representation of certain values and a distortion of the overall picture.
For example, an e-commerce company that has duplicate customer records may overestimate the number of customers. The impact of these issues can be significant, ranging from misleading insights to potentially costly incorrect decisions.
Data Preparation Techniques
Data preparation involves a structured set of techniques to prepare data for analysis. A systematic approach is crucial to ensure that the data is in the correct format, free of errors, and suitable for the intended analysis. The following steps are typically involved:
- Data Validation: This step involves verifying the accuracy and consistency of the data. Techniques include checking for logical errors, verifying data ranges, and ensuring data types are appropriate.
- Data Cleaning: This involves identifying and correcting errors, inconsistencies, and missing values in the data. Methods include handling missing data (e.g., imputation), removing outliers, and correcting incorrect values.
- Data Transformation: This involves converting data into a suitable format for analysis. Techniques include converting data types, aggregating data, and creating new variables. For example, transforming customer demographics from raw data into segmented categories.
- Data Reduction: This involves simplifying the data by reducing the number of variables or observations while preserving the essential information. Methods include dimensionality reduction techniques (e.g., principal component analysis) and sampling.
- Data Integration: This involves combining data from different sources into a single dataset. This step is crucial when dealing with data from various departments or external sources. Ensuring consistency in formats and units is critical for integration.
Data Analysis Techniques
Data analysis is a cornerstone of effective business decision-making. By employing various statistical methods and visualization techniques, businesses can uncover hidden patterns, trends, and insights within their data, leading to improved strategies and outcomes. This section delves into the crucial techniques used to extract meaningful information from data and build predictive models.
Statistical Methods in Business Analytics
Statistical methods are fundamental to business analytics, providing a framework for understanding and interpreting data. These methods range from descriptive statistics, which summarize and describe data, to inferential statistics, which draw conclusions about a population based on a sample.
- Descriptive Statistics: Descriptive statistics are used to summarize and describe the main features of a dataset. Measures like mean, median, mode, standard deviation, and quartiles help understand central tendency, dispersion, and the distribution of data. For example, calculating the average sales revenue for a specific product line reveals trends in sales performance.
- Inferential Statistics: Inferential statistics are used to draw conclusions about a population based on a sample. Techniques like hypothesis testing and confidence intervals allow businesses to make informed decisions about larger datasets without analyzing every single data point. For instance, testing whether a new marketing campaign has a statistically significant impact on sales helps businesses justify the campaign’s effectiveness.
- Regression Analysis: Regression analysis investigates the relationship between a dependent variable and one or more independent variables. Linear regression, a common type, models a linear relationship. For example, understanding how advertising spend impacts sales figures allows for optimizing marketing budgets.
- Time Series Analysis: Time series analysis focuses on data collected over a period of time. Methods like ARIMA models are used to forecast future values based on historical data. Predicting future sales based on seasonal trends is a common application.
Data Visualization for Interpretation
Data visualization tools are essential for effectively communicating insights derived from data analysis. These tools transform complex data into easily digestible charts and graphs, enabling quicker comprehension and identification of patterns.
- Charts and Graphs: Various types of charts and graphs (e.g., bar charts, line graphs, scatter plots, histograms) visually represent data distributions and relationships. These visual representations allow for quick identification of trends, outliers, and correlations. For example, a line graph showing sales over time reveals clear seasonal trends.
- Interactive Dashboards: Interactive dashboards provide dynamic visualizations that allow users to explore data from multiple angles. Users can drill down into specific details and manipulate visualizations to gain deeper insights. An example is a dashboard displaying sales performance across different regions and product categories.
- Data Storytelling: The effective use of data visualization tools is often combined with narrative and storytelling. Explaining the “why” behind the data through visualizations strengthens the communication of insights. For example, a story about declining sales in a specific region could include visuals highlighting sales data, competitor activities, and economic indicators.
Building Predictive Models
Predictive modeling aims to forecast future outcomes based on historical data. Various machine learning algorithms are employed to create these models, enabling businesses to anticipate future trends and make proactive decisions.
- Machine Learning Algorithms: Algorithms like linear regression, decision trees, and support vector machines are used to build predictive models. Each algorithm has strengths and weaknesses, and the choice depends on the specific problem and data characteristics. For example, decision trees are often used for classifying customers based on their purchasing behavior.
- Model Evaluation: Accurate model evaluation is critical. Metrics like accuracy, precision, recall, and F1-score assess the model’s performance. Careful consideration of these metrics ensures the model is reliable and useful for decision-making. For example, a model predicting customer churn might be evaluated based on its ability to identify customers likely to churn.
- Model Deployment: Once a predictive model is developed and validated, it needs to be integrated into business processes. This often involves developing APIs and integrating with existing systems. For example, a model predicting equipment failures could be used to trigger preventative maintenance schedules.
Successful Implementations
Many companies have successfully utilized data analysis techniques to achieve significant improvements. One example is Netflix, which leverages data analysis to personalize recommendations for its users, significantly impacting customer satisfaction and retention. Another example is Amazon, which uses sophisticated algorithms to optimize inventory management and predict customer demand.
Decision-Making Processes
Data-driven decision-making is crucial for businesses seeking to optimize performance and achieve strategic goals. By leveraging business analytics, organizations can transform raw data into actionable insights that inform choices across various functional areas. This section delves into the stages of a robust decision-making process, illustrating how analytical insights can translate into impactful decisions.Effective decision-making is a systematic process, not a haphazard event.
It involves a structured approach that begins with identifying the problem and culminates in evaluating the results. Business analytics plays a pivotal role in each stage, providing the necessary data and insights to support sound judgments.
Stages of a Decision-Making Process
Understanding the various stages of a decision-making process is fundamental to effective data utilization. Each stage plays a crucial role in ensuring that the final decision aligns with organizational objectives.
- Problem Definition: Clearly defining the problem or opportunity is paramount. This involves understanding the context, identifying the key factors, and establishing measurable goals. For example, a declining sales trend necessitates a deeper investigation into potential contributing factors such as changing market preferences or competitor actions.
- Data Collection and Analysis: Gathering relevant data from internal and external sources is essential. This involves selecting appropriate data collection methods, ensuring data quality, and applying analytical techniques to extract meaningful insights. For instance, analyzing customer demographics and purchase history to identify trends and patterns can uncover customer segments with high potential for loyalty programs.
- Alternative Generation: Identifying and evaluating various possible solutions to the problem is crucial. This stage encourages creativity and exploration of different approaches, taking into account available resources and constraints. For example, a marketing team might consider different advertising channels, varying pricing strategies, or implementing new product features to boost sales.
- Evaluation of Alternatives: Assessing the potential impact of each alternative is critical. This involves considering the potential benefits and risks associated with each option, including financial implications, operational constraints, and market response. For instance, comparing the projected ROI for different marketing campaigns or assessing the potential impact of a new product launch on existing product lines.
- Decision Selection: Choosing the optimal solution based on the evaluation of alternatives is the next logical step. This involves considering factors like feasibility, alignment with organizational goals, and potential for success. For example, selecting the marketing campaign with the highest projected ROI or the product launch with the most promising market reception.
- Implementation: Putting the chosen solution into action requires meticulous planning and execution. This involves allocating resources, defining responsibilities, and establishing timelines. For instance, allocating budget, assigning personnel, and developing a comprehensive rollout plan for a new product launch.
- Monitoring and Evaluation: Continuously monitoring the effects of the decision and evaluating its success is vital. This includes tracking key performance indicators (KPIs) and making necessary adjustments. For example, regularly monitoring sales figures and customer feedback to gauge the success of a marketing campaign and adjusting strategies as needed.
Examples of Decisions Benefiting from Business Analytics
Business analytics can be applied to a wide range of decisions across various business functions.
- Pricing Strategies: Analyzing historical sales data, competitor pricing, and market trends to determine optimal pricing strategies that maximize profitability. For example, identifying price elasticity of demand to adjust pricing in response to market fluctuations.
- Product Development: Using customer feedback and market research data to identify unmet needs and develop new products that meet consumer demands. For example, analyzing customer reviews and social media trends to identify product features that resonate with customers.
- Marketing Campaigns: Optimizing marketing budgets and campaign effectiveness by analyzing customer responses and campaign performance data. For example, segmenting customers based on demographics and preferences to tailor targeted advertising campaigns.
Translating Analytical Insights into Actionable Decisions
Effective decision-making hinges on the ability to translate analytical insights into tangible actions. This requires a clear understanding of the business context and a willingness to adapt strategies based on data-driven findings.
- Data Visualization: Presenting data in a clear and concise manner through charts, graphs, and dashboards to facilitate understanding and communication. This can include interactive dashboards for real-time monitoring of key metrics.
- Collaboration: Involving stakeholders across different departments in the decision-making process to ensure alignment and buy-in. For example, integrating sales, marketing, and operations teams to collaborate on data analysis and decision-making.
- Action Planning: Developing specific and measurable action plans based on the analytical insights. This includes defining roles, responsibilities, timelines, and metrics for tracking progress. For instance, creating a roadmap for implementing a new pricing strategy that Artikels the steps, timelines, and metrics for success.
Flow Chart: Data-Driven Decision-Making Process
[Start] --> Problem Definition --> Data Collection & Analysis --> Alternative Generation --> Evaluation of Alternatives --> Decision Selection --> Implementation --> Monitoring & Evaluation --> [End]
Tools and Technologies: Business Analytics And Decision Making Quiz
Business analytics relies heavily on powerful tools and technologies to effectively collect, process, and analyze data. The right tools can transform raw data into actionable insights, driving better decision-making and ultimately, business success.
From sophisticated software to cloud-based platforms, the landscape of business analytics tools is constantly evolving, offering new possibilities for data-driven strategies.
Popular Software for Business Analytics, Business analytics and decision making quiz
A wide range of software solutions caters to various business analytics needs. These tools offer functionalities ranging from data warehousing and ETL (Extract, Transform, Load) processes to advanced statistical modeling and data visualization. Popular choices include tools like Tableau, Power BI, Qlik Sense, and SAS, each with its own strengths and weaknesses. Tableau, for example, is known for its interactive dashboards and visualizations, while Power BI is often favored for its integration with Microsoft ecosystem tools.
- Tableau: Known for its user-friendly interface and robust visualization capabilities, Tableau excels in creating interactive dashboards and reports. Its drag-and-drop functionality allows for quick data exploration and insightful presentations. Tableau is widely used for data storytelling and business intelligence reporting.
- Power BI: Microsoft’s Power BI is a comprehensive business analytics platform that seamlessly integrates with other Microsoft products. Its strength lies in its extensive data connectivity and its ability to create reports and dashboards that can be shared easily with colleagues.
- Qlik Sense: Qlik Sense stands out with its associative engine, which enables users to connect seemingly disparate data points. This allows for more comprehensive insights and faster discovery of trends and patterns. Its visual exploration capabilities are strong, facilitating self-service analysis.
- SAS: SAS is a mature and powerful platform offering a wide range of statistical modeling and analysis tools. It is particularly valuable for complex statistical analyses, forecasting, and predictive modeling, making it suitable for businesses requiring advanced analytical capabilities.
Data Visualization Tools
Effective data visualization is crucial for extracting meaningful insights from complex datasets. Different tools cater to varying visualization needs, and selecting the right tool is important for presenting data in a way that enhances comprehension and fosters understanding. Tools vary in their capabilities, from basic charts and graphs to interactive dashboards and advanced analytics.
- Comparison of Visualization Tools: Different data visualization tools offer diverse capabilities. Tableau excels in creating interactive dashboards, while Power BI excels in its integration with Microsoft tools. Qlik Sense’s associative engine enables more comprehensive connections between data points. Choosing the right tool depends on specific business needs and desired visualizations.
- Choosing the Right Tool: Factors like the complexity of the data, desired level of interactivity, and integration needs determine the optimal visualization tool. A company analyzing sales trends might favor Tableau’s interactive dashboards, while a company requiring complex statistical modeling would lean toward SAS.
Cloud Computing in Business Analytics
Cloud computing has revolutionized business analytics, offering scalability, cost-effectiveness, and accessibility. Cloud-based platforms allow businesses to store and process massive datasets, enabling more sophisticated analyses and faster insights. Cloud platforms also provide flexibility and scalability, adapting to changing business needs.
- Benefits of Cloud Computing: Cloud platforms like AWS, Azure, and Google Cloud provide cost-effective storage, processing, and analytics services. These services offer scalability and flexibility, allowing businesses to adjust resources based on their needs. The ease of access from anywhere with an internet connection enhances collaboration and efficiency.
- Scalability and Flexibility: Cloud-based solutions scale effortlessly to accommodate increasing data volumes and user demands. This scalability is essential for companies experiencing rapid growth or seasonal fluctuations in data needs. The flexibility of cloud platforms allows for quick deployment and adaptation to changing business needs.
Data Security in Business Analytics
Protecting sensitive business data is paramount in business analytics. Robust security measures are essential to prevent data breaches and maintain compliance with regulations. Protecting data integrity and confidentiality is vital for maintaining trust and avoiding reputational damage.
- Importance of Data Security: Data breaches can lead to significant financial losses, reputational damage, and legal repercussions. Implementing strong data security measures protects sensitive business information, ensuring compliance with industry regulations like GDPR and CCPA. Data security safeguards the integrity and confidentiality of the data.
- Implementing Security Measures: Implementing robust data encryption, access controls, and regular security audits are crucial for safeguarding data. Data loss prevention (DLP) technologies can help prevent sensitive information from leaving the organization. Regular security training for employees can also reduce the risk of data breaches.
Case Studies and Examples
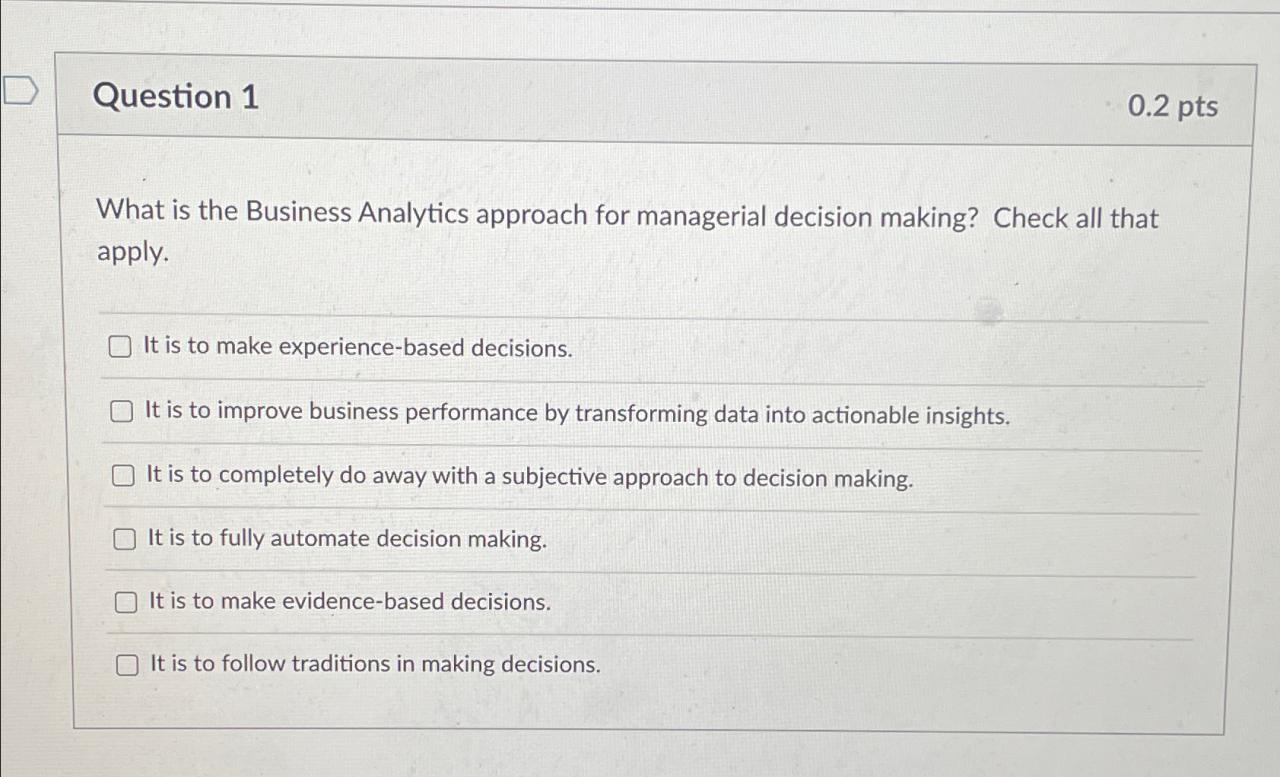
Unleashing the Power of Data: Business Analytics in Action
Business analytics isn’t just a buzzword; it’s a powerful tool transforming how companies operate and make decisions. From optimizing supply chains to predicting customer behavior, data-driven insights are changing the game across various sectors. This section dives deep into real-world examples of how companies are leveraging analytics to enhance their decision-making processes and achieve remarkable results.
A Case Study: Netflix’s Personalized Recommendations
Netflix’s success is intricately linked to its sophisticated recommendation engine. By analyzing vast amounts of user data, including viewing history, ratings, and preferences, Netflix creates highly personalized suggestions. This system, powered by business analytics, significantly improves user engagement and retention. The engine learns user tastes over time, dynamically adjusting recommendations. The result is a streaming service that keeps users coming back for more, showcasing the power of data to cultivate customer loyalty.
Industry Applications of Business Analytics
Data analysis isn’t confined to a single sector. Across various industries, companies are employing analytics to improve operations, enhance customer experience, and increase profitability. This section Artikels how different sectors are using business analytics.
- Retail: Retailers use analytics to understand customer purchasing patterns, optimize inventory levels, and personalize marketing campaigns. For example, Target successfully used data to predict pregnancies, allowing them to tailor marketing to expectant mothers, demonstrating how predictive analytics can lead to targeted and effective campaigns.
- Finance: Financial institutions utilize analytics to detect fraudulent activities, manage risk, and personalize financial products. Credit card companies employ sophisticated algorithms to identify and prevent fraudulent transactions, a vital application of business analytics in the financial sector.
- Healthcare: Healthcare providers utilize analytics to improve patient outcomes, optimize resource allocation, and personalize treatment plans. For example, hospitals use data analysis to identify patients at high risk of complications, allowing proactive intervention and better patient care. This highlights the significant impact data can have on patient well-being.
- Manufacturing: Manufacturing companies use analytics to optimize production processes, predict equipment failures, and improve supply chain efficiency. Predictive maintenance, based on historical data, helps manufacturing companies reduce downtime and maintenance costs, showcasing how business analytics improves operational efficiency.
Impact of Data-Driven Decisions
The impact of incorporating data-driven decisions into business strategies is substantial. Businesses that embrace data-driven approaches experience increased profitability, enhanced operational efficiency, and improved customer satisfaction. The shift towards data-centric decision-making allows companies to anticipate trends, respond to market changes, and proactively adapt to evolving customer needs.
Industry Application Table
The table below illustrates various industry applications of business analytics and showcases successful implementation examples.
Industry | Application | Success Story Example |
---|---|---|
Retail | Demand Forecasting, Inventory Optimization, Personalized Recommendations | Walmart’s use of data to optimize inventory and predict demand, leading to significant cost savings and improved efficiency. |
Finance | Fraud Detection, Risk Management, Investment Strategies | Credit card companies using machine learning algorithms to detect fraudulent transactions, reducing losses and improving security. |
Healthcare | Patient Outcomes, Resource Allocation, Personalized Treatment | Hospitals using data analytics to identify high-risk patients, enabling early intervention and improved patient outcomes. |
Manufacturing | Predictive Maintenance, Supply Chain Optimization, Production Efficiency | Manufacturing companies using predictive analytics to anticipate equipment failures, reducing downtime and maintenance costs. |
Ethical Considerations
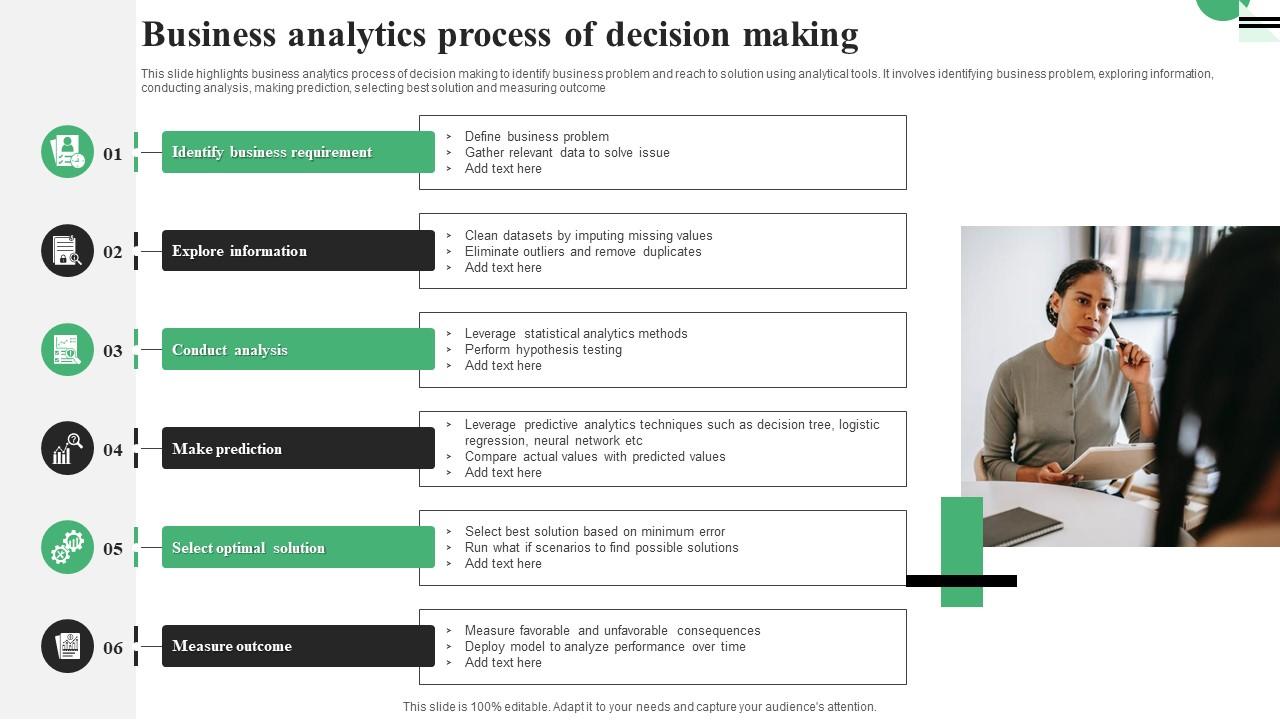
Data analysis, while powerful, comes with significant ethical responsibilities. Using data ethically is crucial for building trust, avoiding harm, and ensuring fairness in business decisions. The ethical use of data in business analytics requires careful consideration of potential biases, privacy concerns, and the broader societal impact of these analyses.
Data analysis techniques, if not applied ethically, can lead to unintended consequences. From perpetuating societal biases to violating individual privacy, unethical data practices can erode trust and damage reputations. A strong ethical framework is essential to ensure responsible data handling throughout the entire analytical process.
Ethical Implications of Using Data in Business Analytics
The ethical implications of using data in business analytics encompass a broad spectrum of concerns. These range from ensuring fairness and transparency in algorithms to safeguarding the privacy of individuals whose data is being analyzed. The potential for bias in data sets and algorithms is a key ethical consideration, as is the potential for misinterpretation or misuse of the results.
Importance of Data Privacy and Security
Protecting the privacy and security of data is paramount. Robust data governance policies, including access controls, encryption, and secure storage procedures, are essential. Organizations must adhere to relevant regulations such as GDPR and CCPA to maintain compliance and build trust with customers.
Addressing Bias in Data Analysis
Identifying and mitigating bias in data sets and algorithms is crucial. Analyzing data for patterns of discrimination or inequality can help uncover hidden biases. Techniques for detecting and addressing bias include diverse representation in data collection, careful consideration of variable selection, and employing algorithms that are less prone to bias. Recognizing and rectifying bias is essential to ensure fairness and prevent unintended consequences in business decisions.
For instance, if a company’s customer service chatbot is trained on a dataset with a significant gender imbalance, it might display biased responses, potentially leading to negative experiences for customers of a certain gender. Careful attention to these factors can prevent such situations.
Examples of Ethical Dilemmas in Business Analytics
Numerous ethical dilemmas can arise in business analytics. For example, using customer data to create targeted advertising campaigns can raise concerns about manipulation and informed consent. Determining the appropriate use of predictive modeling for credit scoring, hiring, or even risk assessment can lead to debates about fairness and accountability. Another significant ethical concern is the potential for discriminatory outcomes in algorithmic decision-making.
Future Trends in Business Analytics
The landscape of business analytics is constantly evolving, driven by technological advancements and the ever-increasing volume of data generated. Understanding these emerging trends is crucial for businesses to adapt, optimize operations, and gain a competitive edge. This evolution requires a proactive approach to learning and adaptation, ensuring organizations are not left behind.
Businesses are increasingly reliant on data-driven insights to make informed decisions. From predicting customer behavior to optimizing supply chains, analytics is transforming how companies operate. These trends are not just about collecting more data; they’re about extracting meaningful information and using it to drive tangible results.
Emerging Trends in Business Analytics
The field of business analytics is rapidly evolving, incorporating new technologies and methodologies. Key emerging trends include the increasing use of artificial intelligence (AI) and machine learning (ML), the explosion of big data, and the growing importance of analytical skills in the workforce. These trends collectively reshape how businesses approach decision-making and operational efficiency.
- Artificial Intelligence (AI) and Machine Learning (ML): AI and ML algorithms are becoming increasingly sophisticated, enabling businesses to automate tasks, identify patterns, and predict outcomes with greater accuracy. For example, AI-powered chatbots are handling customer service inquiries, while ML models are predicting equipment failures to optimize maintenance schedules. This automation not only increases efficiency but also frees up human resources to focus on higher-level tasks.
- Big Data Analytics: The sheer volume of data generated by businesses and customers is exploding, creating unprecedented opportunities for insights. Big data analytics tools are being employed to analyze vast datasets, uncovering hidden patterns and trends that traditional methods might miss. Companies are leveraging this data to personalize customer experiences, optimize pricing strategies, and improve operational efficiency. For instance, retail companies use big data to predict demand fluctuations, leading to optimized inventory management and reduced waste.
- The Importance of Analytical Skills in the Future Workforce: The demand for professionals with strong analytical skills is rapidly increasing. Companies are actively seeking individuals capable of extracting insights from data, interpreting results, and applying them to real-world business problems. This includes professionals in diverse roles, from data scientists to marketing analysts to financial analysts. The future workforce needs a strong foundation in analytical thinking, critical evaluation, and data visualization to thrive in this data-driven environment.
Impact of AI and Machine Learning
The integration of AI and ML into business analytics is revolutionizing various sectors. AI-powered systems can analyze vast amounts of data to identify complex patterns, trends, and anomalies that might otherwise go unnoticed. This capability empowers businesses to make more accurate predictions and more informed decisions. These systems can also automate routine tasks, allowing human analysts to focus on higher-level strategic initiatives.
- Enhanced Decision-Making: AI and ML algorithms can analyze data in ways humans cannot, leading to more accurate and insightful predictions. This allows for more effective strategic planning and resource allocation.
- Automation of Tasks: AI can automate many data-processing and analysis tasks, increasing efficiency and reducing human error.
- Improved Customer Insights: AI can analyze customer data to identify preferences, behaviors, and potential issues, allowing for personalized marketing campaigns and improved customer service.
Big Data Transforming Decision-Making
Big data is no longer a futuristic concept but a tangible force reshaping how businesses operate. By analyzing massive datasets, businesses can identify previously unseen patterns, predict future trends, and make more accurate, data-driven decisions. This transformation is particularly evident in areas like supply chain management, marketing, and risk assessment.
- Improved Supply Chain Management: Big data analytics can be used to optimize inventory levels, predict demand fluctuations, and streamline logistics, resulting in cost savings and increased efficiency.
- Personalized Marketing: Big data can help businesses tailor their marketing campaigns to individual customer preferences, resulting in higher conversion rates and improved customer engagement.
- Enhanced Risk Assessment: Big data analytics can identify potential risks and vulnerabilities, allowing businesses to take proactive measures to mitigate them.
Final Summary
In conclusion, the business analytics and decision making quiz provides a robust framework for understanding the critical role of data in modern business. By mastering the concepts, techniques, and tools presented, you’ll be well-equipped to make data-driven decisions that enhance business performance and drive success. The future of business is deeply intertwined with the ability to leverage data effectively, and this quiz serves as a valuable stepping stone towards that future.