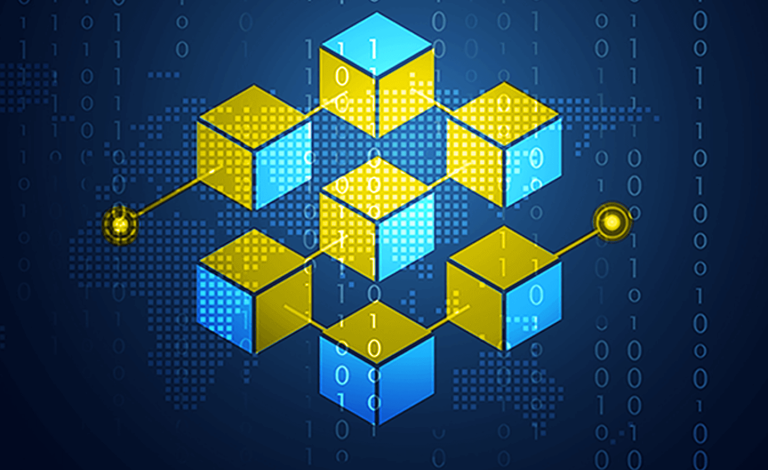
Ethical Questions About Big Data Navigating the Future
Ethical questions about big data are increasingly prominent as data collection and analysis become more pervasive. This exploration delves into the complex issues surrounding privacy, bias, accountability, and the overall societal impact of this powerful technology. From the collection and use of personal data to the potential for bias in algorithms, the ethical considerations are multifaceted and demand careful consideration.
This blog post examines the critical ethical dimensions of big data, addressing concerns ranging from data security and algorithmic fairness to societal implications and data ownership. We’ll explore how data governance frameworks, privacy regulations, and bias detection methods can help mitigate potential harms and ensure responsible big data practices.
Privacy and Data Security
Big data applications are revolutionizing various sectors, from healthcare to finance. However, this powerful technology raises crucial concerns about personal privacy and data security. The vast amounts of data collected, stored, and analyzed necessitate robust measures to protect individual information and prevent misuse. This discussion delves into the complexities of data privacy in the context of big data, examining collection methods, anonymization techniques, encryption, regulations, and governance frameworks.The collection of personal data in big data applications is pervasive.
For instance, online retailers track customer browsing history to personalize recommendations, social media platforms gather user interactions to tailor content feeds, and healthcare providers collect patient data for diagnosis and treatment. These practices, while offering numerous benefits, raise significant concerns about how this data is used and protected.
Personal Data Collection and Usage
Personal data is collected through a variety of means in big data applications. Web browsing history, app usage, purchase records, and social media interactions are just a few examples. These data points, when combined and analyzed, can reveal detailed profiles of individuals, potentially revealing sensitive information about their preferences, habits, and even health conditions. Companies often use this data for targeted advertising, personalized services, and market research.
However, this data utilization must be conducted ethically and transparently, respecting user privacy.
Data Anonymization and Its Limitations
Data anonymization aims to remove personally identifiable information (PII) from datasets while retaining the data’s analytical value. Techniques like pseudonymization, where identifying information is replaced with unique identifiers, and generalization, where specific data points are aggregated into broader categories, are frequently employed. However, these methods are not foolproof. Sophisticated data linkage techniques, such as matching anonymized data with publicly available information, can potentially re-identify individuals, negating the anonymity efforts.
This underscores the need for robust data security measures beyond simple anonymization.
Data Encryption and Access Control
Data encryption converts readable data into an unreadable format, rendering it inaccessible to unauthorized individuals. Robust encryption algorithms are crucial for protecting sensitive information during transmission and storage. Access control mechanisms, like user authentication and authorization, restrict access to data based on predefined roles and permissions. These measures help limit the potential for data breaches and unauthorized use.
Privacy Regulations and Their Impact
Various privacy regulations, such as the General Data Protection Regulation (GDPR) in Europe and the California Consumer Privacy Act (CCPA) in the US, are designed to safeguard individual data rights. These regulations impose strict requirements on data collection, use, and storage, influencing big data practices. Companies must comply with these regulations to avoid legal repercussions and maintain public trust.
Data Governance Framework for Privacy
A robust data governance framework is essential to ensure ethical data practices in big data systems. This framework should encompass data policies, access controls, data quality procedures, and regular audits to identify and mitigate potential risks. It should also prioritize user transparency and consent, ensuring individuals are aware of how their data is being collected and utilized.
Potential Vulnerabilities and Mitigation Strategies
Big data systems are susceptible to various vulnerabilities, including data breaches, unauthorized access, and malicious attacks. Data breaches can expose sensitive information, leading to significant financial and reputational damage. Mitigation strategies include robust security measures, regular security audits, and incident response plans.
Comparison of Data Security Measures
Measure | Description | Effectiveness | Implementation Challenges |
---|---|---|---|
Data Encryption | Converting data into an unreadable format using encryption algorithms. | High, if implemented correctly. | Requires expertise in encryption algorithms and key management. |
Access Control | Restricting access to data based on predefined roles and permissions. | High, if implemented correctly. | Maintaining accurate and up-to-date access controls. |
Anonymization | Removing personally identifiable information from data. | Variable, dependent on the sophistication of re-identification techniques. | Difficult to guarantee complete anonymity and maintain data utility. |
Bias and Fairness in Algorithms
Big data algorithms, while powerful tools, can reflect and amplify societal biases present in the data they’re trained on. This can lead to unfair or discriminatory outcomes for certain groups. Understanding these biases and developing methods to mitigate them is crucial for responsible big data use. Uncovering and addressing these biases is not just an academic exercise; it’s a vital step toward creating fairer and more equitable systems.Algorithms trained on biased data can perpetuate and even exacerbate existing societal inequalities.
This is particularly concerning when these algorithms are used to make critical decisions about individuals, such as loan applications, hiring processes, or even criminal justice evaluations. The consequences of biased algorithms can have a lasting and disproportionate impact on marginalized communities.
Potential Biases in Big Data Algorithms
Data used to train algorithms often reflects existing societal inequalities. For instance, if historical data used to predict future loan applications disproportionately shows defaults among minority groups, the algorithm may learn to discriminate against those groups, even if no explicit bias is coded into the algorithm itself. This learned bias, stemming from historical data, can result in unfair outcomes.
Algorithmic bias is not always intentional; it can emerge subtly from the inherent biases within the training data.
Impact of Data Representation on Societal Biases
The representation of different groups in the data significantly influences the algorithm’s output. If the data predominantly features one demographic, the algorithm may perform well for that group but poorly for others. This uneven representation in the dataset can lead to a misrepresentation of the characteristics and needs of underrepresented groups, leading to unfair or inaccurate results. Algorithms trained on incomplete or biased datasets can perpetuate existing societal inequalities, leading to unfair or discriminatory outcomes.
Methods for Detecting and Mitigating Bias
Detecting and mitigating bias in machine learning models requires a multi-faceted approach. Techniques include examining the data for patterns of disparity across different groups, comparing algorithm outputs to human assessments, and utilizing fairness-aware algorithms.
- Data Analysis and Auditing: Careful examination of the data for disparities in representation across different groups is essential. For example, analyzing the demographic breakdown of the training data for loan applications can reveal whether certain groups are underrepresented. Identifying these imbalances is the first step in addressing bias.
- Fairness-Aware Algorithms: These algorithms are designed to explicitly consider fairness criteria during the training process. For example, some methods aim to minimize disparities in the model’s predictions for different demographic groups. This proactive approach can lead to more equitable outcomes.
- Bias Detection Tools: Specialized tools can identify patterns of bias in the data and the algorithm’s output. These tools can analyze the model’s predictions for different groups and identify potential disparities.
Ethical Implications of Using Big Data for Decisions
Using big data to make decisions about individuals raises significant ethical concerns. The potential for unfair or discriminatory outcomes underscores the need for transparency, accountability, and careful consideration of the potential consequences. These algorithms can perpetuate harmful stereotypes and lead to further marginalization of specific groups.
Examples of Unfair Algorithmic Use
Numerous instances illustrate how algorithms have been used unfairly in the past. One example involves loan applications where algorithms have been shown to discriminate against minority groups. Another example is the use of facial recognition technology, where algorithms have demonstrated higher error rates for individuals with darker skin tones. The historical use of such algorithms in criminal justice has also led to disproportionate outcomes.
Comparison of Bias Detection and Mitigation Techniques, Ethical questions about big data
Technique | Description | Advantages | Disadvantages |
---|---|---|---|
Data Analysis and Auditing | Identifying patterns of disparity in data representation. | Simple to implement, readily available tools. | May not identify all forms of bias, can be time-consuming. |
Fairness-Aware Algorithms | Explicitly considering fairness criteria during training. | Can produce fairer outcomes. | Requires specialized knowledge, potentially complex to implement. |
Bias Detection Tools | Identifying patterns of bias in the data and algorithm output. | Automated identification of potential issues. | May require specialized expertise to interpret results, may not capture all nuances of bias. |
Accountability and Transparency
Big data systems, while powerful, often operate in a “black box” fashion. Understanding how decisions are reached within these complex systems is crucial for ensuring fairness, mitigating bias, and building trust. This opacity can lead to serious consequences, especially when decisions have significant impacts on individuals or society. Accountability and transparency are paramount in addressing these concerns.Establishing clear lines of accountability and ensuring transparency in big data systems are critical for building trust and fostering responsible development and use.
The sheer volume and complexity of data make it challenging to trace the origins of decisions and identify who is responsible when things go wrong. Furthermore, the need for robust explanations and clear communication of the processes involved is essential for understanding and managing the potential biases or errors embedded within algorithms.
Challenges in Establishing Accountability
The complexity of big data systems poses significant challenges in establishing accountability. Tracing the origins of decisions within intricate algorithms and data pipelines can be difficult. The sheer volume of data and the interconnectedness of systems make it hard to pinpoint the source of a problem or the individual or entity responsible. This lack of traceability can lead to a diffusion of responsibility, making it challenging to hold anyone accountable for errors or negative outcomes.
Moreover, the involvement of multiple actors, including data providers, algorithm developers, and system users, adds another layer of complexity.
Importance of Transparency
Transparency in big data systems is vital for both users and stakeholders. Understanding how decisions are made fosters trust and empowers users to challenge or question outcomes. Transparent systems allow stakeholders to assess the validity of results, identify potential biases, and make informed decisions about the use and implications of the data. A lack of transparency can erode public trust and lead to resistance or even rejection of big data applications.
Examples of Negative Consequences from Lack of Transparency
A prime example of the negative consequences of opaque big data systems is the use of predictive policing algorithms. Without transparency, it’s difficult to assess whether these algorithms are unfairly targeting specific demographics. This lack of transparency can perpetuate existing biases and lead to discriminatory outcomes. Similarly, loan applications or hiring decisions based on opaque algorithms can perpetuate historical biases or exclude qualified candidates based on factors not easily understood.
These examples highlight the potential for significant harm when big data systems operate without transparency.
Improving the Explainability of Big Data Algorithms
Improving the explainability of big data algorithms is a crucial step towards fostering trust and accountability. Techniques like model-agnostic explanations and rule-based systems can help to provide insights into the decision-making processes. These approaches aim to translate the complex workings of algorithms into understandable and interpretable outputs, making it easier for users and stakeholders to understand the rationale behind specific decisions.
By enabling more transparent insights, these techniques facilitate greater accountability and mitigate the risk of unfair or discriminatory outcomes.
Role of Human Oversight in Big Data Applications
Human oversight plays a critical role in ensuring accountability and transparency in big data applications. Human review of outputs, monitoring of algorithms, and auditing of data pipelines can help identify and mitigate biases or errors. Human judgment can provide a crucial layer of scrutiny, ensuring that the decisions made by algorithms align with ethical principles and societal values.
Human oversight is particularly important when dealing with high-stakes decisions that have significant impacts on individuals or communities.
Best Practices for Ensuring Transparency and Accountability
- Data Provenance Tracking: Detailed records of data sources, transformations, and usage are crucial. This allows for a clear audit trail, enabling the identification of potential biases or errors.
- Algorithm Documentation: Comprehensive documentation of algorithms, including their inputs, outputs, and decision-making processes, is essential. This allows for a deeper understanding of the algorithms and their potential implications.
- User-Friendly Interfaces: Designing user interfaces that explain the reasoning behind decisions made by algorithms empowers users to challenge outcomes and understand the context of those decisions.
- Independent Audits: Regular audits by independent parties can assess the fairness and transparency of big data systems. These audits can identify vulnerabilities and areas for improvement.
- Ethical Guidelines: Implementing ethical guidelines for the development and use of big data systems is crucial for ensuring responsible practices and minimizing potential harm.
Impact on Society and Individuals
Big data’s pervasive influence on society is undeniable, impacting everything from healthcare and education to employment and social control. While it offers immense potential for progress, its use raises complex ethical questions that demand careful consideration. This exploration delves into the multifaceted impacts of big data on individuals and society, examining both the potential benefits and the inherent risks.The sheer volume of data generated daily by individuals and organizations presents a double-edged sword.
Harnessing this data for positive societal change requires a delicate balance between utilizing its potential for good and mitigating its potential for harm. This involves addressing concerns about privacy, bias, and accountability, but also recognizing the profound impact big data has on our lives.
Potential Societal Impacts: Economic Inequality and Job Displacement
The rise of big data-driven technologies has the potential to exacerbate existing economic inequalities. Algorithms trained on historical data, reflecting existing societal biases, can perpetuate and amplify these disparities. For instance, if an algorithm used for loan applications disproportionately denies loans to people from marginalized communities based on their historical data, it can perpetuate economic disadvantage across generations.
Furthermore, automation driven by big data could lead to job displacement in various sectors, requiring workforce retraining and adaptation to new economic realities.
Ethical Dilemmas Surrounding Social Control
The use of big data for social control raises significant ethical concerns. Surveillance technologies, powered by sophisticated algorithms, can be used to monitor individuals’ activities, potentially infringing on their privacy and freedom. This includes tracking individuals’ movements, online activities, and even their social interactions. The power to analyze and predict behavior raises serious questions about the extent to which such technologies should be used and regulated.
Examples of Big Data’s Use for Societal Benefits
Big data has the potential to revolutionize various sectors, including healthcare. Analyzing patient data can lead to more accurate diagnoses, personalized treatment plans, and improved disease prevention strategies. For instance, predictive modeling based on patient data can help identify individuals at high risk of developing certain diseases, enabling early intervention and reducing the impact of the disease. Similarly, big data is used to optimize traffic flow, improving efficiency and reducing congestion.
Predicting Human Behavior: Risks and Benefits
Predictive analytics based on big data can be used to forecast various societal trends and individual behaviors. This includes predicting crime rates, identifying potential security threats, and understanding consumer preferences. While this capability can lead to more effective interventions and preventative measures, it also carries potential risks. For example, relying too heavily on predictions based on limited data can lead to biased or inaccurate outcomes.
Furthermore, the potential for misuse, such as targeted advertising or social manipulation, requires careful consideration and regulation.
Impact on Education and Personal Development
Big data can enhance educational experiences by tailoring learning materials and methods to individual needs. Analyzing student performance data can identify areas where students require additional support, allowing for personalized learning interventions. However, the potential for data misuse in education settings, such as perpetuating existing inequalities in access to resources, needs careful consideration. It’s crucial to ensure that big data is used ethically and responsibly to promote equity and foster personal growth.
Impacts on Different Social Groups
The impact of big data on different social groups can vary significantly. Certain groups may experience disproportionate benefits or drawbacks. For instance, access to the technologies and resources needed to harness big data may be unevenly distributed, creating a digital divide. Furthermore, pre-existing biases in datasets can lead to discriminatory outcomes for specific social groups. Understanding these potential disparities is essential for mitigating the negative impacts of big data on different segments of society.
Summary Table of Potential Impacts of Big Data
Area | Potential Impacts | Positive Impacts | Negative Impacts |
---|---|---|---|
Healthcare | Improved diagnostics, personalized treatment, disease prevention | Reduced healthcare costs, increased life expectancy, improved patient outcomes | Data privacy concerns, potential for bias in algorithms, digital divide in healthcare access |
Education | Personalized learning, improved educational outcomes, targeted interventions | Increased access to quality education, better student outcomes, tailored learning experiences | Potential for perpetuating existing inequalities, data privacy concerns, lack of equity in access to resources |
Employment | Automation of tasks, creation of new roles | Increased efficiency, reduced costs, new job opportunities in data-related fields | Job displacement in certain sectors, widening income inequality, potential for bias in algorithmic hiring |
Data Ownership and Control
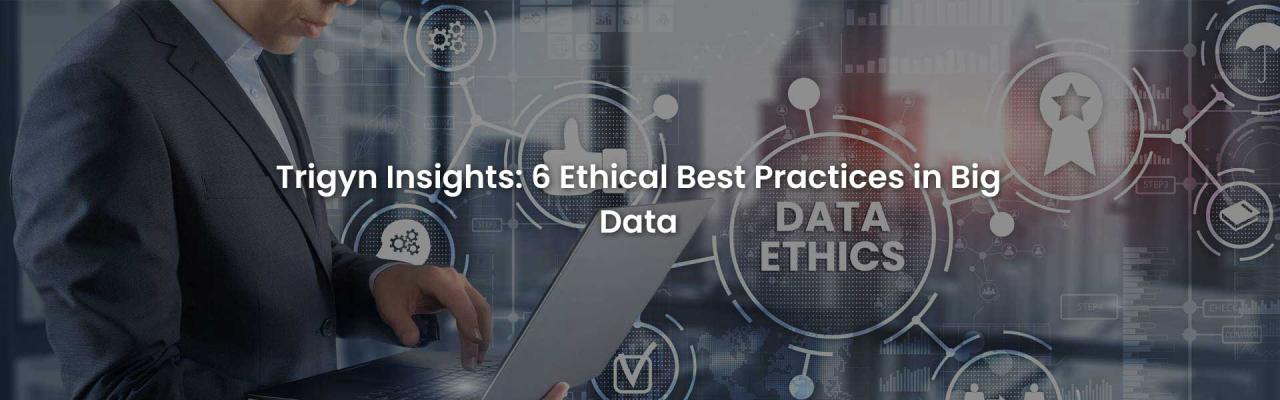
Data ownership in the context of big data is a complex issue, raising crucial questions about who controls the data, how it is used, and what rights individuals have over their personal information. The sheer volume, velocity, and variety of data in big data systems amplify the need for clear guidelines and mechanisms to protect individuals’ rights and ensure responsible data handling.
This becomes particularly challenging in collaborative projects, where multiple parties have access to and potentially different interests in the data.The fundamental concept of data ownership hinges on the idea of individual control over their personal information. This control extends to the ability to understand how their data is being used, to challenge any perceived misuse, and to request the deletion or modification of their data.
The implications for big data systems are significant, demanding careful consideration and implementation of policies and mechanisms to uphold individual rights.
Data Ownership in Collaborative Projects
Determining data ownership in collaborative projects is often fraught with difficulties. Multiple entities may contribute to the data, each with varying levels of control and potential conflicting interests. Agreements outlining data usage, access rights, and responsibilities are crucial but can be challenging to negotiate and enforce. For example, a research project involving multiple institutions might face disagreements on who owns the research data generated by their collective effort.
User Control over Data
User control over their data in big data systems is essential for maintaining trust and fostering responsible data practices. Individuals should have the right to access, correct, and delete their data, and to understand how it is being used. This control extends to the ability to understand the algorithms used to process their data and challenge any potential bias or unfairness.
Users should be informed about the data collected, how it will be used, and what potential consequences there are.
Examples of Exercising Data Control
Individuals can exercise control over their data in various ways. They can review and update their personal information in online profiles, opt-out of data collection in certain contexts, or demand data deletion. Data portability initiatives allow individuals to move their data from one service provider to another, granting them greater control over their information. Further, individuals can utilize data anonymization and encryption tools to safeguard their data from unauthorized access.
Recommendations for Improving Data Ownership and Control
Developing clear and comprehensive data ownership policies and frameworks is crucial for maintaining control and transparency. These policies should be easily accessible and understandable for all stakeholders. Establishing robust mechanisms for data governance and oversight is equally important. This may involve independent audits, data protection officers, and ethical review boards. Furthermore, fostering transparency through clear data usage policies and providing user-friendly tools for data access and control are vital steps.
Different Approaches to Data Ownership and Access
Different approaches to data ownership and access are employed across various sectors. In healthcare, patient data is often subject to strict privacy regulations, while in the financial sector, data security and access are paramount. In academic research, data sharing protocols and ethical review boards play a vital role in safeguarding data integrity and ownership. These varied contexts highlight the need for flexible and context-specific approaches to data ownership and control.
Data Ownership Models in Different Contexts
Different sectors and industries have developed specific data ownership models tailored to their needs. For example, in the financial sector, data ownership is often tied to the entity that holds the legal right to the data, while in the healthcare sector, data ownership often rests with the patient. It is crucial to recognize that these models may differ, necessitating specific and contextualized policies to ensure data ownership and control.
Data Collection and Use in Specific Domains
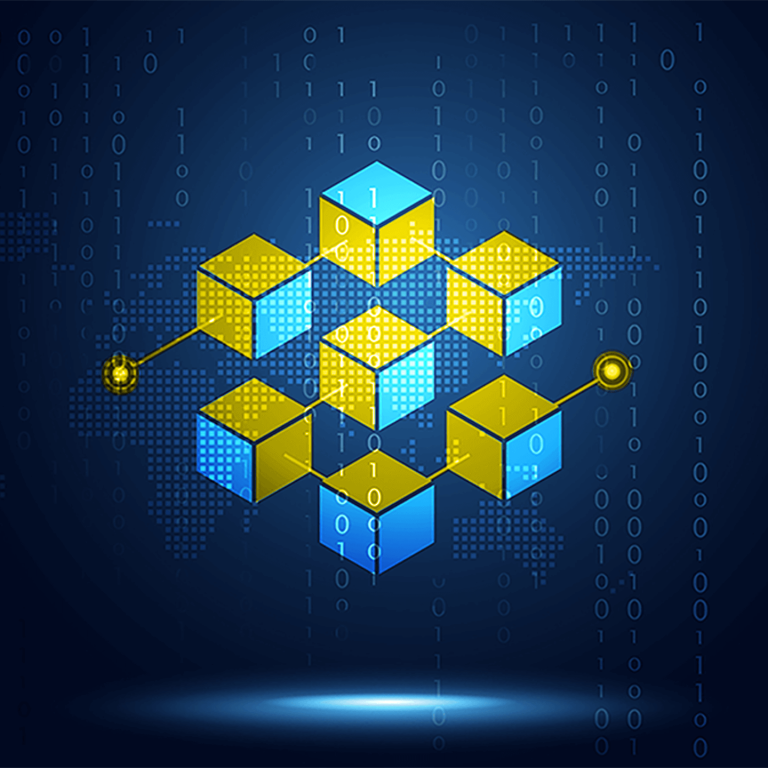
Big data is transforming various sectors, from healthcare to finance and beyond. While the potential benefits are significant, ethical considerations must be paramount. The responsible collection, analysis, and application of this massive dataset are crucial to avoid unintended consequences and ensure fairness and transparency. This exploration delves into the ethical implications of big data in specific domains.The ethical application of big data hinges on careful consideration of the unique challenges and opportunities presented by each sector.
A nuanced understanding of the potential harms alongside the potential benefits is essential to navigate the complex terrain of big data responsibly.
Healthcare
The use of big data in healthcare promises personalized medicine and improved patient outcomes. However, several ethical concerns must be addressed. Data privacy is paramount, requiring robust security measures to protect sensitive patient information. Informed consent is essential for the use of patient data in research and treatment. Potential biases in diagnostic tools derived from data sets reflecting existing societal biases could lead to disparities in care.
Data privacy, informed consent, and potential biases in diagnostic tools are critical ethical considerations in healthcare’s use of big data.
Finance
Big data is revolutionizing financial services, enabling more precise risk assessment and personalized financial products. Algorithmic bias in lending decisions or investment strategies can perpetuate existing inequalities and exclude marginalized communities. Financial inclusion and consumer protection are paramount. The ethical application of big data in finance must prioritize transparency and accountability.
Algorithmic bias, financial inclusion, and consumer protection are key ethical considerations in big data applications within finance.
Criminal Justice
Big data is being used in criminal justice, particularly in predictive policing. However, concerns regarding bias in algorithms, due process, and potential for discrimination remain. The potential for misinterpreting data, leading to wrongful arrests or convictions, is a serious concern. The ethical use of big data in criminal justice requires rigorous scrutiny and safeguards against discriminatory outcomes.
Bias in predictive policing, due process concerns, and potential for discrimination are serious ethical challenges in the application of big data in criminal justice.
Marketing and Advertising
Big data plays a significant role in marketing and advertising, allowing for targeted campaigns and personalized experiences. However, the potential for manipulation, privacy violations, and exploitation raises ethical concerns. The line between personalized recommendations and manipulative tactics must be clearly defined and enforced. Transparency and informed consent are crucial to ensure ethical marketing practices.
Manipulation, privacy violations, and the potential for exploitation are ethical concerns in the use of big data in marketing and advertising.
Successful Applications and Ethical Considerations
Several examples showcase successful big data applications across various domains, highlighting the importance of ethical considerations.
- In healthcare, predictive models using big data can identify individuals at high risk for certain diseases, allowing for preventative measures and timely interventions. However, this must be accompanied by stringent safeguards for patient data privacy.
- In finance, big data analytics can identify fraudulent transactions more efficiently, safeguarding financial institutions and consumers. However, the algorithms used must be rigorously tested for bias to ensure equitable access to financial services.
- In marketing, personalized recommendations based on big data can enhance customer experiences and drive sales. However, the potential for manipulation and privacy violations necessitates clear guidelines and user control over data usage.
Outcome Summary
In conclusion, navigating the ethical landscape of big data requires a multifaceted approach. Balancing the potential benefits of this technology with the inherent risks necessitates ongoing dialogue, robust regulations, and a commitment to transparency and accountability. By acknowledging and addressing the ethical dilemmas surrounding big data, we can strive towards a future where its transformative power is harnessed responsibly and equitably.
Clarifying Questions: Ethical Questions About Big Data
What are some examples of how personal data is used in big data applications?
Personal data is used in numerous big data applications, including targeted advertising, personalized recommendations, and credit scoring. This data can also be used to develop predictive models for various purposes, from healthcare diagnostics to risk assessment.
How can bias be detected and mitigated in machine learning models?
Bias detection techniques involve analyzing data for patterns and disparities that might reflect societal biases. Mitigation methods include re-training models on more diverse datasets, incorporating fairness constraints during model development, and employing techniques like adversarial debiasing.
What are the potential societal impacts of big data, both positive and negative?
Big data can lead to advancements in healthcare, personalized education, and more efficient services. However, it also raises concerns about job displacement, economic inequality, and the potential for social control. The ethical considerations necessitate careful planning and responsible deployment.
How can data ownership and control be improved in big data systems?
Improving data ownership and control involves empowering individuals with greater control over their data, providing clear guidelines for data usage, and implementing mechanisms for data access and consent. Transparency and user-friendly interfaces are essential components.